Data Collection Geared Toward Translational Medicine
Blog: Clinical and Translational
This practice is lab- and data-driven, aspiring to make a bench-to-bedside approach that’ll efficiently develop therapeutic strategies. It identifies biomarkers that can then be used to inform the patient’s molecular profiles and disease etiologies. Biomarkers can include blood sugar levels that identify patients with diabetes, or certain gene mutations that can signal a patient’s risk of developing cancer.
Translational medicine’s use of establishing molecular profiles has been beneficial in creating drugs that are specialized to target specific pathways based on patient diagnoses. This approach, when compared to one-size-fits-all drug production, creates fewer side effects with better results. Translational medicine can be an effective method when done well, with financial benefits on top of health achievements. That said, it’s a data-heavy activity. Here’s an overview of working with data in translational medicine:
Data Collection
From the lab to treatment, there will be ample information collected throughout the process. For successful drug development, all this data must be sorted and analyzed; to make this more effective, data should be responsibly collected from the start with a standardized and efficient practice.
Guidelines for data collection include:
• Collecting enough samples that you can establish statistical significance
• Using the same clinical samples across the entire population
• Using data models with datasets from various sources
• Making sure data is clean and well-curated enough for cross-study analysis
Not only is quality data useful for developing effective drugs, it can also be used retroactively to determine why some drugs weren’t working. For example, the initial compound in a drug for treating non-small cell lung cancer was initially an ineffective treatment for immune responses in autoimmune diseases. Now the drug, Keytruda, is useful for an entirely different purpose than intended.
Data Simplification
The data analysis that follows large-scale genome products can end up fragmented during the process. The creation of many analysis pipelines and informational silos can make it difficult for scientists and clinicians to collaborate.
To be effective, this all needs to be sorted and broken down. A good initial step is making sure that the data is accessible across the board so that even non-experts, or teams, can look at the data, analyze it, and apply their biological understandings. Implementing strategies to streamline data access can save time.
Scalable data management and accessible data tools are vital for translational medicine to succeed, and with that in use, patients can expect to receive valuable, effective drugs.
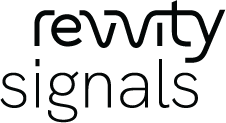
Revvity Signals Software
Revvity Signals Software solutions empower scientists and decision-makers to gain critical insights from data analytics, accelerating informed decisions.