Powering Innovation: Unifying Data and AI to Transform Drug Discovery
How Signals One with AI Enhancement Empowers Researchers
Introduction
Wide deployment of artificial intelligence (AI) is unlocking new efficiencies in research, but it is only as effective as workflows and data allow it to be. As with any computational technology, high-quality data is crucial for achieving optimal results. Barriers to successful AI use in research include data that is not AI-ready, a lack of direct integration of AI into research workflows, and security concerns that may arise from moving data from one system to another. Research software solutions that effectively integrate AI insights and automation into existing workflows can address these obstacles, enhancing and streamlining research.
Enhancing Research with AI
As research complexity and the volume of data increase, coordination often decreases. Knowledge becomes siloed. For example, antibody discovery teams may use vastly different internal processes, software, or manual workflows than bioinformatics teams, leading to fragmented communication and process bottlenecks. The increasing volume of data means that many departments generate analysis backlogs, even before considering requests and coordination with other departments, adding time and effort needed to identify and advance candidates. Streamlining routine analysis and coordination within and among teams can help overcome these challenges.
AI has the potential to revolutionize research and discovery by augmenting menial and routine tasks, freeing scientists to focus on big-picture questions, clear analysis backlogs, and develop cures faster. AI can dig deep into data, surfacing richer insights and making far-reaching connections. As research grows more complex, a lack of data transparency, increasing silos, and inconsistent processes slow discovery throughout the lifecycle— effectively coordinating and managing multifaceted data across knowledge centers is essential. A unified scientific software as a service (SaaS) solution like Signals One™ from Revvity Signals can alleviate these bottlenecks, support AI-augmentation and enhancement, and make research programs ready for this revolution.
The Increasing Complexity of Innovation
Since the first approval of a monoclonal antibody in the 1990s, we’ve seen an explosion in biotherapeutic modalities, including antibody-drug conjugates (ADCs), bispecific antibodies (bsAbs), cell and gene therapies (CGTs).1–3 These new modalities allow entirely new ways to fight disease, but are increasingly complex to develop. This complexity demands a new type of solution that can unite diverse areas of knowledge. Signals One is designed for this complex environment: it provides an end-to-end workflow solution for data capture and collaboration, processing, and decision analytics that harmonize this complexity and enable organizations to deliver candidates and insights faster.
Small-molecule drugs dominated pharmaceutical development for decades, with relatively straightforward development paths and manufacturing processes. However, they have limitations and cannot solve every disease-related problem. Biologics introduced entirely new challenges. Advancements in peptide and protein engineering, extending beyond monoclonal antibodies to create bespoke biotherapeutics, further expanded therapeutic options but required even deeper cross-disciplinary collaboration. For example, ADCs combine the targeting specificity of antibodies with the potency of small molecules and require collaboration between chemistry, cell biology, immunology, and regulatory teams.4 Other complex modalities require similar interdepartmental collaboration to drive progress.
The pace of cell, gene, and nucleic acid therapies is expected to grow, especially with the global response to COVID-19 highlighting the potential for RNA-based therapeutics. Already in 2025, we’ve seen the first FDA approvals for an encapsulated cell therapy for ophthalmology5 and the first mesenchymal stem cell therapy.6 Chimeric antigen receptor (CAR) cell therapies have also become more common, with more approvals and indications, and are now being explored in diseases beyond oncology.1,3 These therapies work in complex biological environments and require even deeper collaboration among more expert knowledge centers. As this trend in drug development continues, developing the next cures will require new insights and ways of integrating diverse expertise.
High-throughput research driven by more predictive analytics and research automation
Traditional high-throughput screening is like trying to find a few needles in hundreds of haystacks. To make high-throughput screening smarter, researchers are increasingly turning to structured, ontology-aware assays. Plug-in assay ontologies, augmented with AI and predictive analytics, are streamlining this process. Assays that are well-defined and ontologically aware enhance data interoperability, accessibility, and retrieval,7 helping researchers discover unknown relationships that may predict which candidates are most likely to succeed. However, effective assay repository design requires integrated, high-quality, and ontologically aware data—a key capability of Signals One.
Siloed systems, common in biopharma and large research organizations, are antithetical to integration. Signals One helps bridge data across knowledge centers and business units to transform data sharing from packaged hand-offs into continuous data streams. This integrated, all-in-one workflow simplifies the complexity of multimodal drug and biotherapeutic development through solution-based approaches that integrate AI to make data sharing less laborious and more intuitive.
More data integration and department collaboration over complex datasets accelerate insights and drug discovery
The AI-augmented research world is already delivering breakthroughs. Bimekizumab, an antibody targeting IL-17A and IL-17F, is the first monoclonal antibody designed by AI and approved by the FDA.8 It was designed using AI trained on high-quality experimental data from hundreds of candidates, and it was able to provide insights to solve a development problem—dual specificity for two closely related cytokines involved in autoimmunity—that the researchers couldn’t solve on their own.8,9 This is just the beginning: in 2023, there were 67 AI-discovered molecules in clinical trials compared with only 7 in 2018.10
Collaboration between knowledge centers and departments often means waiting for specialists, like bioinformaticians, to become available to analyze datasets. Many of these analyses are routine, so this data queuing presents a significant rate-limiting step in research. Packaging data for a handoff to another department also takes time away from analysis and other research tasks. Signals One helps eliminate these bottlenecks by centralizing asset data, improving accessibility, reducing duplicative work, and leaning into the strengths of AI as an assistant, allowing researchers in all knowledge centers to focus on strategy, discovery, and innovation.
Signals One spans the design-make-test-decide cycle in early drug discovery
Signals One easily enables teams of researchers to design, make, test, and decide in the drug discovery process. ADC development requires a pharmacologist to generate new antibodies, assay scientists to screen for candidate antibodies, and chemistry teams to screen small molecule payloads and develop linkers. Signals One simplifies collaboration across these disciplines. It incorporates sophisticated data processing workflows that encompass a range of common analytical tasks—including in vitro curve fitting, in vivo data analysis, and group comparisons—and ensures intuitive analytics through standardized analysis pipelines. Even in routine antibody development, challenges include selecting high-affinity hits in early screening that ultimately fail due to unfavorable biophysical properties and a lack of visibility into molecular structures that present risks, both within and across teams. Signals One can identify liabilities like: hydrophobicity, charge asymmetry, and CDR length early, allowing teams to prioritize candidates based on these developability metrics before advancing candidates doomed to fail.
AI insights are only as good as the data. By adhering to FAIR principles (Findable, Accessible, Interoperable, Reusable), Signals One helps ensure data quality and make data AI-ready from the start. Standardized assays and data analysis protocols allow researchers to generate deeper, faster insights by making data comparable and interpretable across experiments. Beyond simply improving modes of collaboration, AI could provide insights across time and departments that individual researchers may not see. Signals One is also powered to streamline data retrieval: never lose track of important data again with AI-powered semantic searches, protocol assistant, and image text recognition.
Conclusion: Simplify Science with Signals One
Signals One empowers researchers to tackle the growing complexity of multimodal drug discovery by enabling collaboration and enhancing workflows with AI assistance. Researchers can spend more time on discovery, creativity, problem-solving, and creating cures, and less time wrangling data and handling disjointed systems. With ontology plug-ins to help contextualize findings and automated visualizations and on-the-fly summaries to make data immediately actionable, researchers can reach conclusions faster and spend less time manually assembling hand-off reports. As a result, teams can spend less time pursuing ineffective options, instead focusing on the best candidates and accelerating them toward clinical trials.
References
1. Jin S, Sun Y, Liang X, et al. Emerging new therapeutic antibody derivatives for cancer treatment. Sig Transduct Target Ther. 2022;7(1):1-28. doi:10.1038/s41392-021-00868-x
2. Pal LB, Bule P, Khan W, Chella N. An overview of the development and preclinical evaluation of antibody–drug conjugates for non-oncological applications. Pharmaceutics. 2023;15(7):1807. doi:10.3390/pharmaceutics15071807
3. Alnefaie A, Albogami S, Asiri Y, et al. Chimeric antigen receptor T-cells: an overview of concepts, applications, limitations, and proposed solutions. Frontiers in Bioengineering and Biotechnology. 2022;10. Accessed August 7, 2023. https://www.frontiersin.org/articles/10.3389/fbioe.2022.797440
4. Labrijn AF, Janmaat ML, Reichert JM, Parren PWHI. Bispecific antibodies: a mechanistic review of the pipeline. Nat Rev Drug Discov. 2019;18(8):585-608. doi:10.1038/s41573-019-0028-1
5. FDA approves revakinagene taroretcel-lwey (ENCELTO) for macular telangiectasia type 2. Ophthalmology Times. March 6, 2025. Accessed April 10, 2025. https://www.ophthalmologytimes.com/view/fda-approves-revakinagene-taroretcel-lwey-encelto-for-macular-telangiectasia-type-2
6. Research C for DE and. FDA approves remestemcel-L-rknd for steroid-refractory acute graft versus host disease in pediatric patients. FDA. Published online December 18, 2024. Accessed April 10, 2025. https://www.fda.gov/drugs/resources-information-approved-drugs/fda-approves-remestemcel-l-rknd-steroid-refractory-acute-graft-versus-host-disease-pediatric
7. Vempati UD, Schürer SC. Development and Applications of the Bioassay Ontology (BAO) to Describe and Categorize High-Throughput Assays. In: Markossian S, Grossman A, Baskir H, et al., eds. Assay Guidance Manual. Eli Lilly & Company and the National Center for Advancing Translational Sciences; 2004. Accessed May 1, 2025. http://www.ncbi.nlm.nih.gov/books/NBK92017/
8. Zhavoronkov A. AI in big pharma: the first antibody designed using AI in the clinic. Forbes. December 21, 2022. Accessed June 10, 2024. https://www.forbes.com/sites/alexzhavoronkov/2022/12/21/ai-in-big-pharma-the-first-antibody-designed-using-ai-in-the-clinic/
9. Callaway E. How generative AI is building better antibodies. Nature. 2023;617(7960):235-235. doi:10.1038/d41586-023-01516-w
10. Jayatunga MK, Ayers M, Bruens L, Jayanth D, Meier C. How successful are AI-discovered drugs in clinical trials? A first analysis and emerging lessons. Drug Discovery Today. 2024;29(6):104009. doi:10.1016/j.drudis.2024.104009
Related Resources
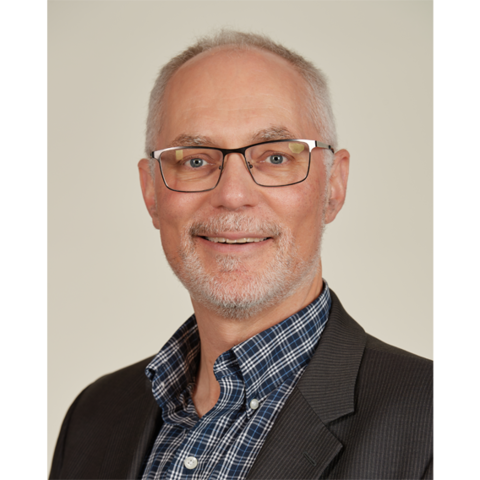
Chris Stumpf
Senior Principal Marketing Professional, Revvity Signals Software, Inc.Chris Stumpf is a Senior Principal Marketing Professional responsible for Marketing Programs at Revvity Signals Software, Inc. Chris has over 20 years of experience in the Analytical Instrumentation and Informatics industry spanning Pharmaceuticals & Life Sciences to Chemicals & Materials. He has a Ph.D. in Analytical Chemistry and Mass Spectrometry from Purdue University.