From Data Overload to Insight: Using AI and ML for Security Trend Prediction
As in nearly every other field, artificial intelligence (AI) – and particularly machine learning (ML) – is revolutionizing the cybersecurity space, allowing systems to defend against new and novel threats like never before possible. The need for these tools is rapidly growing, commensurate with the exponential jump in data generation required in multiple sectors, especially scientific research and development.
Data breaches are increasingly costly and dangerous, and failure to properly protect data can lead to commercial disadvantages, regulatory pressure, loss of reputation, and public embarrassment. Thankfully, the same AI attributes that companies have already been eager to leverage for data management – namely, its ability to quickly and efficiently interrogate, analyze, and correlate data among giant volumes through pattern recognition – is also what makes AI invaluable for securing data.
Those capabilities have made AI and ML algorithms indispensable tools for scientific discovery and biopharma breakthroughs, with the high-throughput capacity needed to model molecular synthesis, analyze integrated data, and improve both internal and external communication. (You can learn more about Revvity Signals’ AI leadership here.)
AI tools can also be designed to do things that older security technologies have not been able to do. In particular, they can uncover trends and make correlations faster and more deeply than manual processes. ML algorithms can be trained to detect patterns without a reliance on external sources to deliver them. These heuristic learning patterns allow for the possibility of refinement unmatched by other automated methods, enabling quick and accurate identification of anomalies and unauthorized access
The most successful AI makes processes more efficient so that personnel can use their time for higher-order tasks. In cybersecurity, that means decreasing the amount of manual data interrogation and correlation required. Fewer manual steps not only shrink the time burden but also reduces the number of errors due to the potential for humans to miss critical cybersecurity correlations.
As a result, skilled specialists can instead get alerts when something occurs outside of normal user and process-usage patterns. Their responsibility becomes triaging and investigating any anomalies, then putting in place remedial actions if a presumed threat is valid.
AI changing the world
ML and other AI techniques have been used in a variety of sectors for some time, including the cybersecurity space and have been delivering true value in data security for decades.
As far back as the early 2000’s, ML algorithms began replacing rules-based systems that needed predefined parameters. Algorithms like these are trained on large data sets, and then deployed to address any unusual activity in user actions and network traffic patterns.
The technology has advanced significantly since those first deployments and can now be used in more sophisticated ways to construct more secure environments. Some of the most powerful approaches use heuristic tools, a behavioral-based approach that allows for rapid decision-making. Heuristic tools leverage AI efficiency and correlation capabilities to do things that more simplistic signature-based technology cannot.
Signature-based tools must start from a known issue, meaning they must be constantly updated – and even if they are, the latest signatures won’t be able to capture the newest threats. This is a particular problem for zero-day vulnerabilities, which are discovered by those looking to exploit a weakness before a vendor even knows it exists. The result is an unacceptable number of “fire drills” and a security system that is inevitably playing catch-up at all times.
This is the key difference between signature-based and heuristic models. Signature-based tools can’t deal with a new or zero-day vulnerability until a risk is identified elsewhere, followed by some period of time for developing the vendor patch, downloading the new signature, and updating the security system.
By contrast, AI tools that analyze behavioral traits create heuristic usage patterns that can help to identify zero-day vulnerabilities that haven’t been identified yet and do not have a fix. ML-based heuristic tools themselves identify normal usage patterns, and then build a model of behavior. When they identify patterns that fall outside typical usage, the heuristic systems flag it through an alert.
As a result, even zero-day vulnerabilities that have never been encountered can be flagged. ML-based platforms like Revvity Signals’ Cloud Detection Response can detect such threats in real-time.
Another advantage is that the more data a heuristic AI tool is exposed to, the more it learns and develops, meaning the model is constantly evolving to protect against new threats. The result is more advanced preparation and less need for fire drills.
Fighting fire with fire
Heuristic tools for threat modeling and malware identification are just some of the many AI security solutions that can help protect a system, including anomaly detection and bot attack prevention.
It should come as no surprise that AI is well-suited to counter AI-based security threats. One of the biggest data security risks today comes from cybercriminals who themselves are using AI for increasingly sophisticated attacks. According to the Federal Bureau of Investigation, attackers are using AI to automate existing tactics, as well as to augment their speed and potential scale.
But just as AI is only one tool in the arsenal for attackers, multiple tools are needed to protect your data and systems as well. A good defense should have multiple layers, like a virtual fortress. Revvity Signals uses a Zero Trust model that addresses security at several levels, pairing AI with tools like data encryption and strict access controls. The concept is to ensure every possible attack vector is secured, be it data, host, platform, or network.
Implementing a robust cyber-defense starts by identifying risks at every level, then systematically minimizing the security threats. Coordinated plans must be in place for responding to incidents based on foreseeable scenarios. And every layer must be auditable to verify preparedness and regulatory compliance. Finally, no cybersecurity system is complete without the human element, and implementation depends heavily on well-trained personnel to manage technical security controls.
Read more about how Revvity Signals’ software uses AI and many other techniques to protect data like yours here.
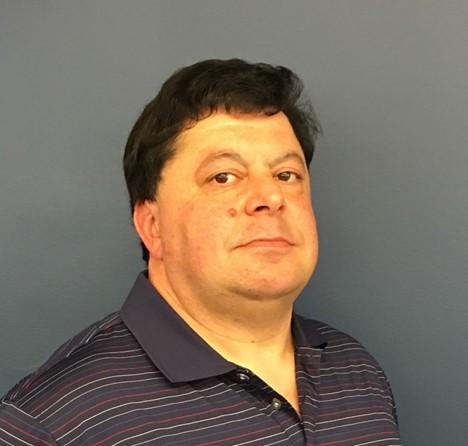
Rick Percuoco
Head of Cloud OperationsRick is the Head of Cloud Operation for Revvity Signals. Rick leads a global team of approximately 40 people and is responsible for CloudOps, SecOps, DevOps, and FinOps for Signals SaaS products. Rick has 10+ years’ experience in Enterprise Cloud platforms, Cloud operations, deployment automation, Cyber Security, Cloud performance and finance cost optimization.