How to Deliver Clinical Data Analytics at Scale to Speed Informed Decisions
How to Deliver Clinical Data Analytics at Scale to Speed Informed Decisions
The data generated during clinical trials is critical and managing it can sometimes feel more complex than the targeted disease! Clinical researchers gather extensive data from each trial participant to ensure the safety and efficacy of new therapeutics.
The result? A data management landscape that is chaotic, cumbersome, time-consuming, and more costly and inefficient than it needs to be.
Often, so much time must be devoted to aggregating, cleaning, and otherwise managing the data that little time is left for generating insights from clinical data – the core of clinical research from which informed study decisions are made.
The challenge is clear: today's clinical researchers need tools for managing large volumes of complex data swiftly and efficiently to speed innovation and informed decisions. The solution is a clinical data science platform that integrates FAIR data principles.
How can data management be streamlined?
By adopting FAIR data principles (FAIR data is Findable, Accessible, Interoperable, and Reusable), we can transform clinical trial data management. This paves the way for faster, more efficient, and accurate data analytics.
While FAIR data principles debuted in 2016, surprisingly little has been done to integrate them into the pharmaceutical industry's best practices and clinical data management systems.
Let’s take a closer look at the FAIR data principles and explore how you can leverage them to streamline your clinical trial data management and decision-making.
Understanding FAIR Data Principles
In case you’re not familiar with FAIR data principles, we thought a quick explanation would be helpful. FAIR data principles provide a robust framework for data management. These principles are not theoretical concepts but practical guidelines that can streamline R&D efforts across industries. For our purposes here, we’ll discuss them in relation to clinical trials.
- Findable: Data should be well-described, identifiable, and searchable. For example, in an oncology trial studying various tumor types, including tumor type in both the trial title and individual patient data ensures that healthcare professionals and investigators can easily locate specific information.
- Accessible: This means data, including metadata and dictionaries, is understandable to both developers and computers. Ideally, accessible data are housed in a secure, user-friendly common space, not scattered across different platforms.
- Interoperable: Data should be integrated and fully harmonized with other data to avoid the pitfalls of siloed information. Siloed data cannot be thoroughly examined, applied to subsequent trials, or indicate red flags that need attention. And that means key insights may be missed by clinicians.
- Reusable: Data should have clear usage licenses and be associated with accurate information, facilitating easy comparison across similar trials.
Applying FAIR data principles to the clinical trial process, from start to final data analysis and reporting, creates efficiencies. This saves your organization time and money and produces better results and deeper insights.
By optimizing FAIR principles through centralized data management platforms, pharmaceutical and biotechnology companies can shift their focus from managing the data to understanding and applying the data.
Centralized data management tools are pivotal in the FAIR data landscape.
A centralized clinical data science platform can deliver self-service, analytics ready data. That same platform combined with an analytics tool like Spotfire can also provide clinical and operational insights that empower your clinical development teams to make informed decisions.
Data management using FAIR data principles can be automated with a data management platform in place. And when the information is centrally located and analysis-ready, it meets compliance requirements and accelerates end-of-study timelines.
Revvity Signals Clinical is a software-as-a-service (SaaS) clinical data science platform that supports the application of FAIR principles. It serves up time relevant analyzable information by generating harmonized and standardized data. Signals Clinical plus Spotfire combines scalable data management with advanced data visualization capabilities for improved complex data analysis.
The platform minimizes manual effort and maximizes the value of data through automated processes. Signals Clinical provides configurable workflows to support data management, clinical data review, and study monitoring activities.
Advanced AI/ML Analytics
In addition, a clinical data science platform can help you deliver strategic analytics such as artificial intelligence (AI) and machine learning (ML). The ability to use machine learning to identify clinical sites at risk of adverse event (AE) underreporting is just one example of the many potential applications of AI in clinical development.
A Machine-Learning (ML) algorithm can be applied to analyze site and patient AE data to predict whether a site is overperforming or, more importantly, underperforming in its AE reporting. This use of machine learning enables sponsors to quickly identify at-risk clinical sites that need closer management to ensure optimal performance.
Signals Clinical also facilitates seamless collaboration across the clinical development team via real-time data sharing and commenting on specific data points. Integrated collaboration enables clinicians to review, query and track the status of line listings and ensure that queries are captured, documented, and can be referenced by study managers. This clinical data science platform leads to better results, deeper insights, time and cost savings, and improved efficiency for future trials.
Revvity Signals Clinical for Future-Ready Clinical Trials
Integrating FAIR principles into your data management system is more than a step toward efficiency; it's a giant leap toward conducting future-ready clinical trials.
With a centralized clinical data science platform like Signals Clinical, your organization can spend less time preparing clinical data for analysis and more time analyzing and applying it.
Explore how Signals Clinical can revolutionize your approach to data management, aligning with the FAIR principles for more effective, compliant, and insightful clinical trials.
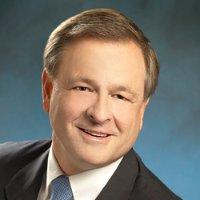
Mark Weadon
Clinical Analytics Product Marketing ManagerMark Weadon has been active in clinical development analytics and visualization for over 20 years. Mark currently serves as the Clinical Analytics Product Marketing Manager at Revvity Signals. Mark has thirteen years of pharmaceutical industry experience at GlaxoSmithKline. Mark pivoted into life sciences product marketing with roles at SAS Institute, IBM, Definitive Healthcare, and Revvity Signals. Mark holds an MBA from Elon University and a BA from Duke University.