AI-Powered Drug Discovery Possibilities | Revvity Signals Software
Antibody research flows in four highly connected yet distinct streams: monoclonal antibodies (mAbs), bispecific antibodies (bsAbs), antibody drug conjugates (ADCs), fragment crystallizables (FC). The first monoclonal antibody was generated in 1975, and around a decade later the FDA issued its first mAb approval for Orthoclone OKT3®, muromonab-CD31, to help prevent rejection in organ transplantation. At the time of writing, the FDA has approved more than 150 mAbs in total for cancer, autoimmune and infectious diseases, inflammatory and other conditions.
Similarly, more than thirty years later in 2017, the FDA approved the first chimeric antigen receptor T cell (CAR-T) therapy,2 and 15 antibody-drug conjugates (ADCs) have been approved to date.3 Next generation bsAbs and multi-specific antibodies offer considerable advantages over mAbs, such as enhanced target engagement, multi-pathway inhibition, personalized treatment, combination therapy optimization, and an improved safety profile.4
Today, the market and the weight of research investment indicates significant change: Sales of the top-selling drugs for 2022 show a roughly 40:60 split between small molecules and larger biologics. In the ten years to 2024, global investment in drug discovery has approximately doubled to around $71 billion, and investment in monoclonal antibodies has approximately tripled to perhaps $10 billion.5
In addition, FDA approvals are increasing: in the decade from 2000, the FDA approved 20 new antibody therapeutics, an average of two approvals each year. In the most-recent ten years, 2013-2023, the FDA issued 99 approvals, almost five times as many – and rising.6
Antibody research divides in favor of non-cancer therapies, 57:42. Around 40% of US adults will be diagnosed with some form of cancer during their lifetime,7 and cancer accounts for approximately 18% of all deaths in the US. Heart disease is actually the number one killer, at 20%. Yet for hereditary angioedema, only lanadelumab has been approved, with garadacimab currently in review and no other cardiac therapies in prospect.8
What you don’t see you don’t know
Prior to the theoretical research and subsequent discovery of monoclonal antibodies, you might argue that collectively we did not know or understand that mAbs, bsAbs, ADCs and FCs even existed. The entire field was effectively out of sight, as an unknown unknown. Diseases previously assumed to be un-druggable and un-investable have become treatable and profitable.
To make the intellectual leap to a new modality and address a disease area that is currently out of sight as an unknown unknown, many companies are turning to AI. By combining AI with vast libraries of existing data and large language models (LLMs), this new machine intelligence offers the potential to uncover entirely new drug discovery landscapes.
Two AI examples spring to mind, AlphaFold and Firefly Bio, which take the same premise and explore very different outcomes.
Developed by Google DeepMind, AlphaFold, now in its third iteration, computationally predicts 3D protein structures. While the results are not always accurate, AlphaFold demonstrates how AI might be able to accelerate candidate selection by orders of magnitude. AlphaFold has published more than 200 million protein structure predictions, including nearly all catalogued proteins.
In contrast to the Google behemoth, Firefly Bio represents the new kid on the block, recently emerging from its stealth mode with a $94 million funding deal. Firefly aims to develop new cancer treatments based on convergence of ADCs and protein degraders (PROTACS) in a novel synthesis called degrader antibody conjugates (DACs).9 Fueled by AI, Firefly asserts that DACs are exactly the kind of new modality that can be explored. By using LLM technology to absorb very large existing datasets, AI can trawl huge volumes of candidates prior to investing time and resources into physical development and manufacture. Vertex Pharmaceuticals also recently announced their intention to use Orum Therapeutics’ dual-precision targeted protein degradation (TPD²®) technology platform to discover targeted conditioning agents for use with their gene editing therapies.10
A fascinating take on how you might combine machine intelligence with human intelligence comes from Dr. Jiye Shi, whose team created bimekizumab, the first and so far possibly the only computationally designed monoclonal antibody: “To me, the real breakthroughs in drug discovery are when different minds come together to see something that we were not able to see individually. By “minds” I mean both human and AI/ML.”11 Bimekizumab not only breaks new ground for treating plaque psoriasis, it addresses a potentially highly profitable marketplace.
Last year, Absci became the first company to create and validate novel antibodies using zero-shot generative AI.12 Zero-shot AI involves designing antibodies to bind to a specific target without relying on data from antibodies that have already been discovered. This approach to drug discovery has the potential to reduce the time for novel therapeutic antibodies to reach the clinic from up to 6 years to around 2 years. With the high-throughput wet lab technology they have developed, Absci report that they can test and validate almost 3 million unique antibody designs per week.
Other companies are following close behind. For example, Genentech is also harnessing AI and machine learning to identify, optimize and design novel antibodies. The recent acquisition of Prescient Design allowed them to integrate a manifold sampler, which allows scientists to make changes to a protein sequence, saving time in antibody design. Genentech hope that the advantages of AI and machine learning will enable them to bring antibody-based therapies to clinical trial more quickly, and they feel that the proof-of-concept phase of using these approaches is nearly over, with real progress beginning to be seen.13,14
The research and development biotechnology company, Evotec, recently used a form of machine learning called a Generative Adversarial Network to generate a phage display library of antibodies. They screened the library to identify novel antibodies which bind to the SARS-CoV-2 spike protein with therapeutically relevant affinity and specificity. Evotec’s in silico engineering approach allows antibody development to be biased towards antibodies with desirable attributes, such as suitability for common manufacturing processes, high stability in long-term storage, low-viscosity for injections, and long elimination half-lives.15
The kicker is that for machine learning approaches to work, we need large, high-quality datasets, and the ability to update those datasets even as the discovery continues. Rejected candidates are every bit as important as positive candidates. Accurate, informative and complete coverage of research will create the secure data foundation that machine learning and AI clearly needs. The difficulty is that it is all too easy to focus on the visible data, yet discovery lies in the invisible areas. Think of icebergs; nearly 90% is out of view, and decisions based only on the 10% might lead to disaster. In the same way that negative clinical trial results provide critically important datapoints yet are often suppressed, do we overlook or ignore the goldmine of data created by discarded compounds?
Where do we go from here?
While AI holds immense promise in the antibody drug discovery field, at the moment it requires compound libraries in which to search for candidate drugs. 16 This means that it has limitations – for example, if it hasn’t been possible to crystallize a transmembrane protein, it won’t be included in the compound library. AI in this context is more like an ‘augmented’ intelligence that supports and complements the research team.
Considerably more verified data is needed to develop AI/ML models, and more advanced ML models need to be designed to improve their predictive power. Further research is also needed into the immunogenicity long-term effects of next-generation mAbs.17 Researchers and developers are actively exploring how to optimize the properties of bsAbs and multi-specific Abs to enhance their stability, pharmacokinetics, and tissue penetration, to minimize their immunogenicity, and to modify them to facilitate large scale manufacturing. The use of AI in this field is an upcoming area of active research.18
Revvity Signals helps organizations to take advantage of AI-ready data within antibody drug discovery. Revvity Signals enables researchers to gain the most out of their data by providing rich context and metadata-tagged datasets that create the essential training foundation for AI/ML endeavors.
Like research itself, no single software solution is likely to be perfect or sufficient to meet the complex demands of todays scientists. Our software is an extension of your scientific capabilities to structure and organize data to help you reveal your data’s deepest insights. Revvity Signal’s commitment is to the journey, helping you to discover new landscapes, new modalities, and new therapies. Recognizing the need that multiple software solutions are required to support today’s complex research needs, Signals Research Suite, from Revvity Signals, includes ChemDraw to infuse chemical drawing with biological intelligence, Signals Notebook to provide unified data management, and Signals Research Suite Data Processing to run AI-driven data analytics and decision support.
If you need more information on how Signals Research Suite can help you accelerate scientific insights, enhance collaboration, and fast-track your drug discovery, please contact us.
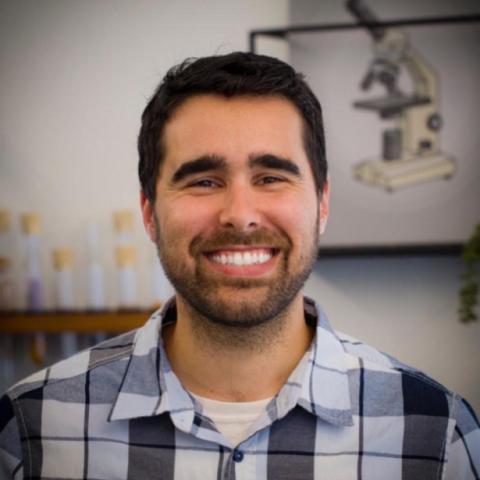
Zev Wisotsky, Ph.D.
Sr. Principal Marketing ManagerZev is a Sr. Principal Marketing Manager for Biologics in the Signals Suite. His scientific training and research background includes neuroscience, biochemistry, molecular biology, and drug discovery. He has spent 7+ years in software in go-to-market teams across industries with a heavy focus on biopharma/biotech R&D.