Lighten Up: Machine Learning Powers Brightfield Revival for Cellular Phenotyping
AI boosts brightfield imaging for fast, label-free cell analysis.
After years in the darkness, machine learning is enabling next level phenotypic insights with brightfield imaging thanks to the groundwork of cell painting and immunofluorescence.
Reversing Course
What does it take for a successful pioneer to change a fundamental factor of its approach?
Recursion Pharmaceuticals has been an undisputed leader in using ML for drug discovery, with multiple AI-enabled programs in the clinic. Its approach is to train algorithms on images of cells demonstrating the phenotypic changes relating to specific genetic or chemical modifications. When it launched in 2013, the company capitalized on a then-new trend in imaging: the use of cell painting for the visual identification of cell components.
Cell painting uses a series of organelle-/ component-specific fluorescent dyes to produce high-contrast images at scale. When cell painting was introduced, it seemed an obvious improvement over the low-fi approach – brightfield imaging, a concept effectively dating back centuries where just a powerful light source shone through cells on a slide. Recursion had used its cell painting-based assay to build AI models that could predict the cause of perturbations to cells and the mechanisms of actions driving them.
But a decade later, Recursion announced it was reversing course.
The Fluorescent Trade-off
While cell painting boosted contrast, the fluorescent dyes came with two particular downsides. The first was the costs: although scalable, the approach still took more steps, labor, time, and money than brightfield imaging. More importantly, the dyes themselves could impact cell behavior, or be cytotoxic or phototoxic. As a result, cells must be fixed before painting, limiting its utility for observing time-bound event. Recursion was excited by the fact that with brightfield, the same cells in the same sample could be used in multiple different assays.
What others had discovered, and what finally changed Recursion’s approach, was the fact that AI doesn’t have the same limitations as human perception, and the difference in contrast had little to no impact on algorithms’ ability to discern the features of a cell. So last year, Recursion announced its transition to brightfield-based phenomics.
Brightfield and AI in High-Content Screening
If a picture is worth a thousand words, what’s a movie worth? The goal of phenotypic imaging has always been to track and measure biologically relevant activity within cells’ natural environment, without bias. The ability to track and watch the progression of time-bound events using time-lapse microscopy opens up all new possibilities for phenotypic observations of cellular dynamics.
So, what can we actually do now with brightfield imaging? We asked Martin Daffertshofer, who is the High-Content Screening Software Product Manager here at Revvity Signals.
“Now that we have tools like deep learning, we can understand brightfield images much, much better, and see all the cellular compartments pretty nicely,” he says. “It starts with a nuclear segmentation, cytoplasm segmentation, and next we are going into subcellular compartments. You can use deep learning in screening application where you do not want to modify a very complex set of parameters beforehand.”
He continues: “The other aspect is it doesn't require too much coding. We learn from scientists, who define their assays based on what they would like to see in the end. That means it's more or less a training aspect rather than a coding aspect. Running it in a seamless workflow will move towards high-content screening, but also to standard applications within cell counting as such.”
The Phenologic.AI Approach for Cell Imaging
Martin and his team have recently released new capabilities it had been developing for Phenologic.AI, a ML pre-trained module within Signals Image Artist, Revvity Signals’ next-generation image analysis and management platform. Phenologic.AI has been pre-trained on thousands of diverse images and tested with 50 different kinds of cell lines – and growing, as his group continues to feed the model more data.
Another plus to Revvity Signals’ approach to AI in imaging is the ability to capture images at frequent intervals. Signals Image Artist is purpose-built to generate, store, and manage large volumes of imaging data. It also helps integrate Phenologic.AI with other tools that can be used for secondary analysis.
The promise of ML and AI in imaging has always been to augment human capabilities, and the recent advances are a stark demonstration of this potential. Though brightfield imaging had fallen out of vogue, AI has unlocked new abilities to answer biological questions we have not been able to ask previously, as there has never been a straightforward experimental approach to do so. And it will undoubtedly lead to new questions that we have yet to think of.
For questions on implementation, we’re here to help. Reach out to the Revvity Signals team today!
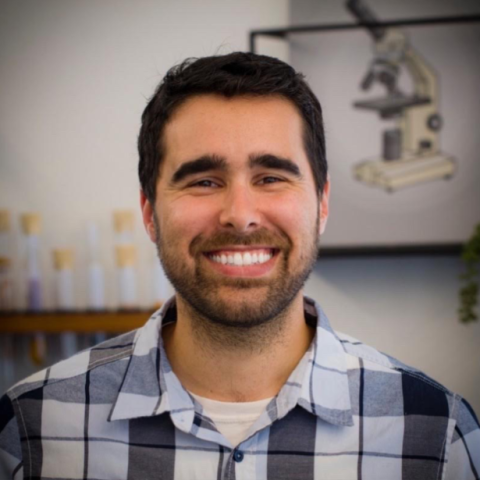
Zev Wisotsky, Ph.D.
Sr. Principal Marketing ManagerZev is a Sr. Principal Marketing Manager for Biologics in the Signals Suite. His scientific training and research background includes neuroscience, biochemistry, molecular biology, and drug discovery. He has spent 7+ years in software in go-to-market teams across industries with a heavy focus on biopharma/biotech R&D.