Phenotypic Drug Discovery with Cell Painting
New molecular entity registrations are on the decline. Eroom's law – which states that it's becoming more expensive and slower to bring new therapeutics to market – continues to hold true since it was postulated in the 1980s.
Things may be changing, however. Better disease stratification, intelligent experimental design, and improved computational biology are helping scientists to overcome Eroom's law. Both computational and digital biology are seeing heavy investment. Phenotypic drug discovery is gaining traction over traditional target-based screening.
Supercharge Your Phenotypic Screening
Cell Painting is a phenotypic screening method that combines cell and computational biology to understand how cells behave under treatment.
It’s a method for profiling multiple phenotypic parameters in cells using fluorescent bio probes to label different cellular compartments. First discussed in 2013, it wasn’t until 2016 that the approach was modified and given the name ‘Cell Painting.’ Cell Painting is a multistep process that helps us understand the effects of chemical compounds, drugs, genes, or other environmental factors. It has been used to:
- Identify new therapeutics.
- Assess human gene function.
- Evaluate environmental toxicants.
Cell Painting offers new opportunities for studying cellular-level mechanisms. The approach has the potential to reduce animal testing, streamline therapeutic testing, and investigate toxins.
How Cell Painting Works: An Overview
Cell Painting gets its name because it stains or “paints” multiple components of a cell such as the nucleus, nucleoli, mitochondria, endoplasmic reticulum, and so forth. Cell Painting is not done in one step, but involves many steps including the following:
- Treatment & Staining: Cells are placed in a microplate, treated, and cell stains added. Usually this is done with automatic robotic systems due to the high volume of plates.
- Image acquisition: Samples are analyzed with high-content optical based screening instruments.
- Feature Extraction: Cell features or phenotypes are converted from images into numbers.
- Downstream Computational Analysis: dimensionality reduction, statistical analyses, such as unsupervised clustering, are typically performed.
The assay involves high-throughput robotics and automation, fluorescent bio probes, image acquisition and analysis, and machine and deep learning tools. The resulting high dimensional data is displayed using tools such as PCA, t-SNE or UMAP to visualize clusters of similar phenotypic profiles and suggest a mechanism of action for unknown compounds.
Diving Deeper in Cell Painting
Cell Painting uses cells seeded into microplates and treated with compounds. The cells are typically treated for 24-96 hours and analyzed using statistical techniques to cluster similar phenotypic profiles.

The Cell Painting assay involves labeling cells with fluorescent probes. Commercially available probes are commonly used to reduce costs and increase throughput. In current Cell Painting experimental design, six fluorescent probes are used to stain eight cell organelles and components.
Images of labeled cells are captured by a high-throughput microscopy system, either sequentially or simultaneously. Spectral overlap of fluorescent probes can be solved through spatial localization in x, y, and z dimensions. Modifications of the Cell Painting probes are made to better characterize a disease state or detection on a High Content Screening (HCS) imaging device. The challenge is handling the narrow separation of the spectral properties of multiple fluorescent dyes.
Cell Painting uses image segmentation to identify unique properties of individual cells that result in feature measurements. These measurements include cell morphology, intensity of probed markers, and dynamics. The features are averaged per well and can be used to measure population and subpopulations of the response – especially in heterogeneous cell models or mixed co-culture systems.
The number of image-derived metrics quickly expands with the number of fluorescent biomarker probes per cellular compartment and any subpopulations. A typical phenotypic screen generates a few dozen measurements, but the full extent of image analysis can easily generate hundreds or thousands of features in a Cell Painting assay.
Cell Painting Challenges
While Cell Painting offers many powerful insights into cellular phenotypes, there are also many informatics challenges due to the large quantity of rich information. Some of the challenges include:
- Image Processing
Preprocessing of Cell Painting images involves several steps such as image normalization, background correction and cell segmentation. - Feature Extraction
Cell Painting generates images, each with several channels, resulting in a high-dimensional dataset. Extracting meaningful features from this dataset can be challenging and requires robust algorithms that can handle the variability in the images. - Data integration Cell Painting generates multiple datasets that need to be integrated for downstream analysis. Integrating data from multiple sources can be challenging.
Signals Image Artist and Signals VitroVivo address these Cell Painting Informatics challenges by converting images to numbers and then finally to assay endpoints as shown:
Signals Image Artist
- Segment: Identify the objects to investigate
- Refine: Adjust the areas of interest
- Quantify: Extract properties out of the image
- Classify: Machine learning to refine classification of cellular populations
Signals VitroVivo
- Dimensionality reduction
- Advanced statistics and unsupervised machine learning for gaining insights.
Cell Painting uses analytical tools, including artificial intelligence or machine learning guidance, to better understand, fingerprint, and interpret data to identify new discoveries. Read the cell painting white paper, learn more about Cell Painting or explore the capabilities of Signals Image Artist and Signals VitroVivo.
Learn more about Revvity Signals solutions spanning the Cell Painting workflow.
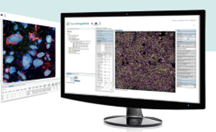
Signals Image Artist supports image data from all major high-content screening and cellular imaging systems. It uses high performance computing and an industry standard object store to provide a scalable, multi-user solution for image analysis and management that can expand with your labs evolving needs.
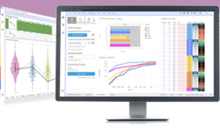
Signals VitroVivo unites assay development, low throughput to ultra-high throughput production assays, High Content Screening, and in vivo studies so users can search across all assay and screening data in a single platform.
Working with Cell Painting data is complex. Sometimes you need expert guidance. The journey from unusable data to actionable insights begins here.
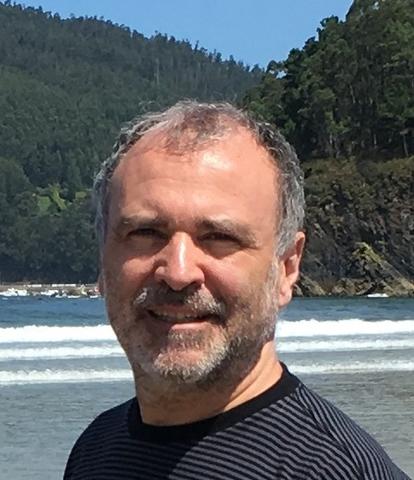
Eduardo Gonzalez-Couto
Product Manager for Signals VitroVivoDr. Gonzalez-Couto is currently responsible for the core screening capabilities of Signals VitroVivo and formerly was product manager for translational medicine, bioinformatics products strategist & manager at Revvity Signals. Previously, he was CSO at Integromics and has more than 25 years of professional experience in bioinformatics gained at GlaxoWellcome, GeneProt, and Siena Biotech. He received his Ph.D. in Molecular Genetics after obtaining a Master in Molecular Biology and graduating in Biochemistry at the University of Geneva, Switzerland.