FEATURED POST
Revvity Signals 2024: Empowering Scientific Innovation and Collaboration
In 2024, Signals ChemDraw, Signals Clinical and Signals Synergy launched. Read how Revvity Signals supports new modalities in drug discovery and research development.
January 7, 2025 Read Blog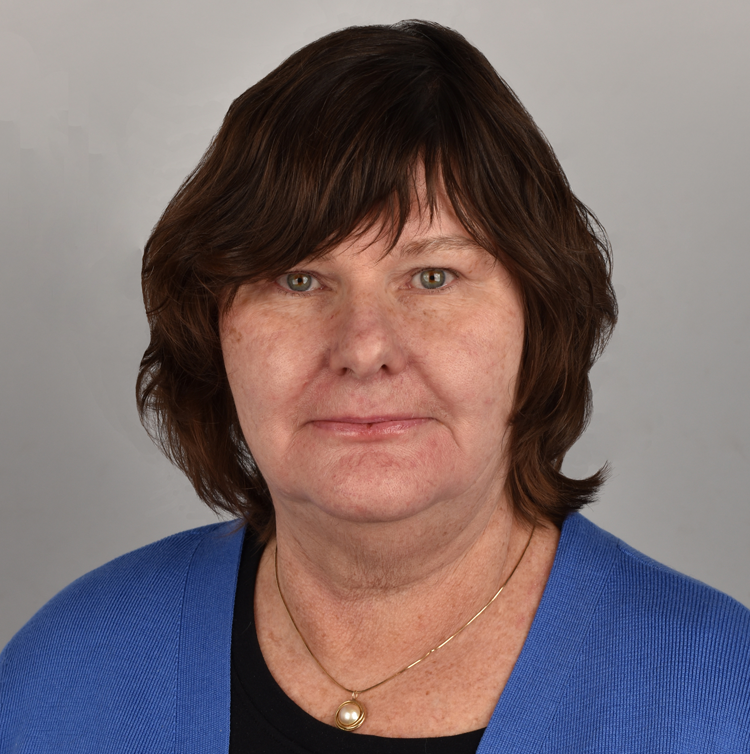
WRITTEN BY:
Mary Donlan, Ph.D.
Executive Director, Product Marketing